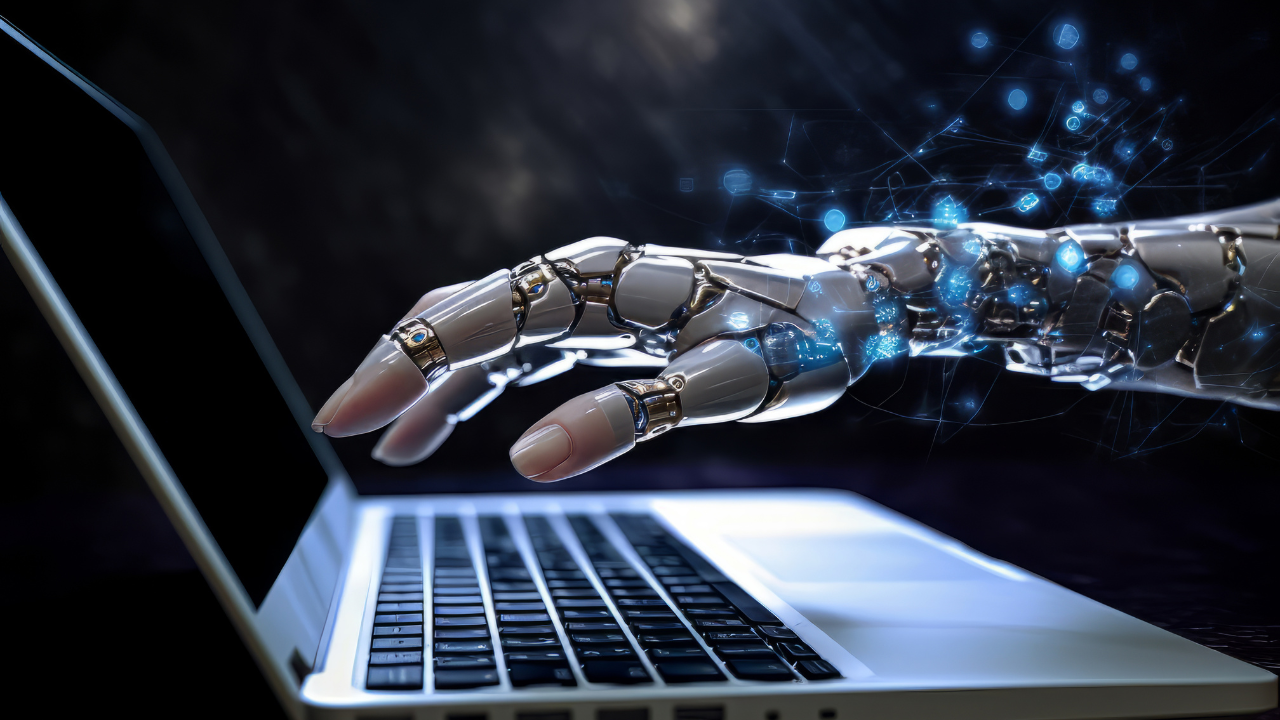
Currently, the distinction between machine learning and artificial intelligence (AI) is becoming increasingly clear. A branch of machine learning and artificial intelligence involves developing algorithms that allow computers to learn and enhance their functionality over time. Conversely, artificial intelligence encompasses a broad range of technologies aimed at creating systems capable of performing tasks that typically require human-like intelligence. This includes understanding natural language, recognizing patterns in data, and making decisions.
Understanding the intricate relationship between machine learning and artificial intelligence (AI) is crucial in today’s technological landscape. This understanding unlocks the full potential of both fields, leveraging their unique strengths to develop intelligent and adaptable systems. By integrating AI algorithms with a broader AI framework, developers and researchers can create, refine, and deploy smart technologies that tackle complex issues and propel advancements across various industries.
This blog post aims to delve deeper into the relationship between machine learning and artificial intelligence. We will explore how these two areas intersect, complement each other, and how their synergy fosters innovation and optimization in modern technology. Furthermore, we will discuss real-world examples, future trends, and ethical considerations surrounding the deployment of intelligent technologies. By the end of this exploration, readers will gain a comprehensive understanding of the symbiotic nature of machine learning and artificial intelligence and their pivotal role in shaping the future of technology and society.
Table of Contents
Understanding Machine Learning
Characteristics of machine learning and its fundamentals
Machine learning, a branch of artificial intelligence, allows computers to learn from data without being explicitly programmed. It encompasses various methods, including supervised learning, where algorithms learn from labeled data to make predictions or classifications. Unsupervised learning, on the other hand, involves algorithms identifying patterns or structures in unlabeled data. Reinforcement learning allows algorithms to learn through trial and error, making decisions based on feedback from interactions with their environment. Together, these methodologies lay the groundwork for machine learning algorithms, enabling computers to gain knowledge and improve their performance over time.
Provide examples of machine learning applications in various industries
Machine learning is revolutionizing industries, transforming operational practices and propelling organizations forward. In healthcare, machine learning algorithms sift through medical imagery, aiding in disease diagnosis and treatment planning. The finance sector employs machine learning for fraud detection, risk management, and optimizing trading strategies. E-commerce platforms utilize machine learning to tailor product recommendations to consumers, based on their browsing and purchasing patterns. Meanwhile, transportation companies are harnessing AI to optimize routes, forecast demand, and advance the development of self-driving vehicles. These examples underscore the diverse applications of machine learning across sectors, illustrating its profound influence on industry norms and outcomes.
Review the work of information, computations, and models in the machine learning process
The data server is key to machine learning, driving the processes of learning and analysis. Having access to sophisticated, crucial data is vital for developing accurate machine learning models. Operations like extraction, sorting, resampling, clustering, and anomaly detection depend on algorithms. These algorithms, including decision trees, support vector machines, neural networks, and deep learning models, are designed for specific data types and challenges. As they process data, these algorithms build models that uncover patterns or relationships, allowing for informed predictions or decisions. The continuous improvement of these models through feedback enhances their accuracy and adaptability. Therefore, the interplay between data, algorithms, and models is fundamental to machine learning, ensuring its effectiveness and flexibility in various domains and applications.
Exploring Artificial Intelligence
Define Artificial Intelligence and its Scope
Artificial Intelligence (AI) stands at the crossroads of software engineering, aspiring to build systems capable of executing tasks typically necessitating human intellect. These tasks span a broad spectrum, including language comprehension, pattern recognition, solving complex challenges, and making basic decisions. AI systems are designed to mimic human cognitive functions such as perception, reasoning, critical thinking, understanding, and language mastery. Covering a diverse array of technologies, methodologies, and practical applications, AI extends its influence from robotics and self-driving cars to virtual assistants and healthcare diagnostics, showcasing its pivotal role in advancing various domains.
The evolution of artificial intelligence from its beginnings to the present
The genesis of artificial intelligence (AI) dates back to the mid-20th century when pioneers in science began exploring the potential for machines to process and exhibit behavioral understanding. Early efforts in AI research focused on symbolic reasoning and rule-based systems, leading to the development of expert systems designed to replicate human expertise in specific domains. Nonetheless, these initial advances were hampered by limitations in processing power and an inability to tackle complex real-world problems effectively. The evolution of AI methodologies in the latter half of the 20th century revolutionized the field, enabling computers to learn from data and, over time, improve their performance autonomously.
Different categories of AI
Artificial intelligence (AI) spans a spectrum of sophistication, from narrow AI to general AI. Narrow AI, often referred to as weak AI, is designed to execute specific tasks within a constrained domain. These AI systems excel in functions like language translation, recommendation engines, image recognition, and gameplay. However, they lack the broader cognitive abilities inherent to human intellect.
Different categories of AI
Figure out how machine learning squeezes into the more extensive field of artificial intelligence
Machine Learning serves as the cornerstone within the broader domain of artificial intelligence, offering a pragmatic approach to developing intelligent systems. Whereas artificial intelligence encompasses a wide array of techniques aimed at emulating human-like intelligence, machine learning focuses specifically on enabling computers to learn from data, thereby enhancing their performance over time. As such, Machine Learning is a crucial component of the AI toolkit, facilitating the creation of intelligent systems that can adapt to new information and environments.
How machine learning strategies add to the development of artificial intelligence
Machine Learning is crucial for enhancing artificial intelligence (AI) capabilities. By learning from data, AI improves in tasks like pattern recognition, navigation, and problem-solving. It enables AI to gain knowledge, understand complex topics, and improve performance autonomously. This process of continuous learning and adaptation drives AI evolution, resulting in more sophisticated and efficient systems.
Difficulties and Restrictions Looked by Both Machine Learning and Artificial Intelligence
Recent years have witnessed significant advancements in Machine Learning and Artificial Intelligence. However, these fields also face several challenges and limitations that slow their progress and widespread adoption.
Data Quality and Quantity
Machine Learning models are fundamentally dependent on data for their training processes, requiring not just vast amounts of it but also ensuring that this data is of high quality. The challenges in meeting these requirements are multifaceted. For instance, biases present in the data can lead to skewed results, undermining the model’s accuracy and fairness. Incomplete datasets, on the other hand, may not provide a comprehensive view necessary for the model to learn effectively, leading to gaps in its knowledge.
Interpretability and Transparency
Artificial Intelligence models, particularly those based on deep learning, lack interpretability, which makes understanding their decision-making processes challenging. This lack of transparency raises concerns about accountability and reliability.
Overfitting and Generalization
Overfitting occurs when a model excels with training data but fails to generalize to new, unseen data. Finding the right balance in model complexity to prevent overfitting while ensuring it can generalize is a fundamental challenge in machine learning.
Ethical and Cultural Implications
Artificial intelligence frameworks, while innovative and transformative, have the potential to perpetuate biases present in their training data, which can lead to unfair and unjust outcomes. This replication of bias can affect decision-making processes in critical areas such as hiring, law enforcement, and loan approvals, perpetuating existing societal inequalities. Therefore, it is of utmost importance to address ethical issues surrounding AI, including concerns about privacy, bias, and discrimination.
Robustness and Security
Artificial intelligence models are vulnerable to adversarial attacks, wherein malicious inputs can skew their predictions. Ensuring resilience and security in AI systems is crucial, particularly in sensitive areas like healthcare and finance.
Computational Assets and Scalability
Deploying complex artificial intelligence (AI) models demands significant computational resources and specialized hardware. Scaling AI systems to process large datasets can become costly and resource-intensive. Addressing these challenges necessitates collaboration among researchers, policymakers, and industry stakeholders to develop ethical, transparent, and robust AI systems that benefit society while minimizing risks.
Pingback: What Is Emerging Technologies - Diverse Nexa